SMART
Science-informed Machine Learning for Accelerating Real-Time Decisions in Subsurface Applications (SMART) Initiative
1/1/2020 – 12/31/2021
GCCC Principal Investigator: Seyyed Hosseini
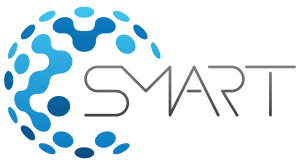
Background
The SMART Initiative is funded by the Department of Energy’s Carbon Storage and Upstream Oil & Gas Programs to transform how people interact with subsurface data by application of science-based machine learning and data analytics. Read more and find partners on DOE’s webpage.
Objective
The objective of this task is to demonstrate an alpha-version interactive platform for dynamically learning how a CO2 storage reservoir performs/behaves for different operational strategies, with an initial emphasis on placement and operation of both injection wells and extraction wells.
Approach
Under this subcontract, UT-BEG will actively participate and contribute to developing Rapid Forecasting Workflows and proof-of-concept demonstrations.
GCCC will identify various types of reservoirs, reservoir properties and operational parameters that are most relevant. In addition, UT-BEG will provide clean datasets based on real field applications that can be used as a working dataset. Furthermore, UT-BEG will implement relevant deep/machine learning tools to the selected synthetic data sets.
Last Updated: June 28, 2021